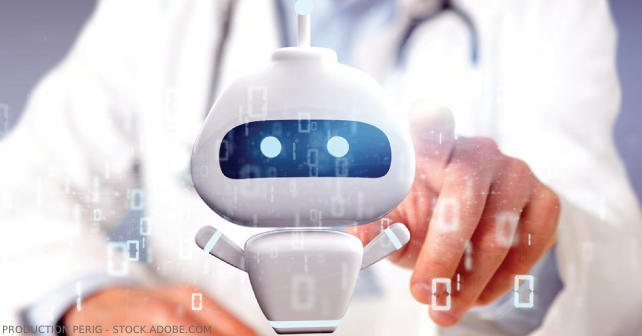
If you’re wondering about the hype with chatbots in medicine, perhaps it’s because they’re nothing new: the first medical chatbot, after all, was developed back in 1964. Using a simple pattern-matching and reflection script entitled DOCTOR, the ELIZA program simulated a Rogerian psychotherapist. Even this basic initial experiment evoked unique responses from those interacting with the software, and a new field of human-computer interaction was born. These natural-language capabilities have evolved over many years, from those found as digital assistants ubiquitous on websites, to the current state-of-the-art ChatGPT, currently based on the generative pre-trained transformer-4 architecture, better known as GPT-4.
Explore This Issue
ACEP Now: Vol 42 – No 06 – June 2023The GPT-4 used in ChatGPT and Bing and its cousins Language Model for Dialogue Applications, or LaMDA, at Google and Large Language Model Meta AI, or LLaMA, at Meta, are examples of large language models, or LLMs. These are neural-network models tuned and trained on vast amounts of data, on the order of hundreds of billions of words) split into smaller components called tokens. These models take prompts (again in tokens) comprised usually of words, numbers, and text annotations, and generate an output based on statistical predictions of the next token in sequence. As all the tokens in sequence are words and parts of words, the form of this output takes the form of coherent sentences. This is similar to the “auto-complete” sometimes seen in word-processing applications, or in text messaging on mobile phones, but dramatically more sophisticated.
The power of being able to prompt using natural language and generate output based on, effectively, near-encyclopedic knowledge of everything is immediately obvious and easy to demonstrate. One of the most-publicized demonstrations by the team responsible for the development of GPT-4 involves its performances on medical licensing examinations.1 The team responsible for GPT-4 obtained a set of questions from the United States Medical Licensing Examination (USMLE) for Step 1, 2 and 3, and prompted the GPT-4 LLM with the questions as text-only prompts precisely as they would appear in a live examination. The model was asked to simply supply a response indicating the correct answer from the multiple-choice set presented. Whereas previous versions of GPT were unable to pass the medical examinations, GPT-4 demonstrated scores of approximately 85 percent correct on each Step of USMLE, well above the passing threshold.
If the response to such an achievement is “that’s all well and good, but basic science and general medical knowledge ain’t brain surgery,” that line of investigation has already been covered as well.2 A team from Brown University obtained the Self-Assessment Neurosurgery (SANS) from the American Board of Neurological Surgery and administered its content to GPT-4. The average performance of a neurosurgical trainee on this examination is 73 percent, with a passing threshold of 69 percent. The GPT-4 model scored 83.4 percent, obtaining a passing score and comfortably exceeding the average human performance. Given these examples, it seems likely LLMs can competently guess the correct answers with sufficient accuracy to pass any multiple-choice medical examination.
In the New England Journal of Medicine, members of the team from Microsoft Research and OpenAI give a high-level overview of performance on question banks, but also explore additional avenues for LLM applications.3 In one example provided, a GPT-4-based chatbot provides answers to a simulated use case with questions regarding medications for diabetes. Unsurprisingly, considering the large body of content available regarding the diabetes medication metformin, GPT-4 is able to provide a coherent and accurate informational response to a simple initial query. However, the example also demonstrates a common problem with LLMs termed “hallucinations,” in which the output is grossly incorrect. In subsequent follow-up prompts in the example provided, the GPT-4-based chatbot claims to have a master’s degree in public health, as well as personal family experience with diabetes. These spurious generative examples demonstrate some of the limitations of LLMs; specifically that they do not rely upon underlying reasoning and insight.
The effects of “hallucinations” become more apparent when these models are prompted to add rigor and explanation to their answers. A project based on a previous version of GPT, GPT-3.5, prompted the LLM to provide answers to a set of medical questions and provided them to experts to rate their content.4 Out of 20 questions and responses, the experts identified five major and seven minor factual errors. More interestingly, the authors also asked GPT-3.5 to supply references for their medical explanations. The LLM generated 59 supporting references, 70 percent of which the authors determined were outright fabrications. These fabrications were usually composed in the proper journal citation format, sometimes used author names from published articles relevant to the question prompt, and otherwise outwardly appeared legitimate, but simple verification steps revealed them to be fiction. While this experiment was performed using GPT-3.5, these errors persist in GPT-4.
Another proposed application for LLMs involves transcription and summarization services to reduce administrative overhead. The aforementioned New England Journal of Medicine article was also authored in part by representatives from Nuance Communications, in Burlington, Mass., highlighting this future product offering. In their example, the software passively listens to, and creates a transcript of, a conversation between a clinician and a patient. Following the patient encounter, the software then summarizes the encounter in the form of a doctor’s note. Generally speaking, the LLM creates a narrative matching the input, but also hallucinates details not present in the original encounter. The example provided illustrates both the potential and current limitations of the technology, presently requiring careful review to ensure spurious extrapolations have not been inserted into the medical documentation.
From these applications, it is clear these models in their present form have real potential pitfalls. Simply predicting the next entry in a sequence of words is divorced from the “real” intelligence and critical appraisal of a medical decision-making process. The current state of the art is in a sort of so-called “uncanny valley,” in which the realism is near-human, but remains problematic enough to become disconcertingly unnatural. Likewise, the danger of confidently imperfect natural language output is immediately obvious, requiring vigilant error-checking and potentially negating some of the advantages in time saved. Only an expert-level clinician may be capable of identifying minor inaccuracies in clinical guidance, while identifying transcription and coding errors may prove practically impossible, given the content necessary for review for validation.
These concerns aside, however, it is worth noting the leap from GPT-3.5 to GPT-4 required only a few months of additional development, while adding a significant leap in performance. The teams developing and tuning these models are acutely aware of the issues and obstacles present in their models. Future versions are likely to have greater accuracy and error-checking abilities, as well as improved domain-specific generative abilities. Just a few months ago, these models were hardly part of the public consciousness, and these are just the first initial steps in determining their potential applications and the refinements necessary. Even if these models are not quite yet ready for use today, their future use to augment decision making and productivity is inevitable.
Dr. Radecki (@emlitofnote) is an emergency physician and informatician with Christchurch Hospital in Christchurch, New Zealand. He is the Annals of Emergency Medicine podcast co-host and Journal Club editor.
References
- Nori H, et al. Capabilities of GPT-4 on medical challenge problems. Cornell University arXiv website. doi: https://doi.org/10.48550/arXiv.2303.13375. Published March 20, 2023. Last revised April 12, 2023. Accessed May 15, 2023.
- Ali R, Tang O, et al. Performance of ChatGPT and GPT-4 on neurosurgery written board examinations. medRxiv website. https://doi.org/10.1101/2023.03.25.23287743. Published March 29, 2023. Accessed May 15, 2023.
- Haug CJ, Drazen JM. Artificial intelligence and machine learning in clinical medicine 2023. N Engl J Med. 2023;388(13):1201-1208.
- Gravel J, et al. Learning to fake it: Limited responses and fabricated references provided by ChatGPT for medical questions. medRxiv website. https://doi.org/10.1101/2023.03.16.23286914. Published March 24, 2023. Accessed May 15, 2023.
Pages: 1 2 3 | Multi-Page
No Responses to “Dr. Chatbot Will See You Now”